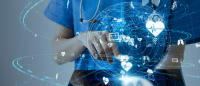
Q&A: Artificial Intelligence and Nursing
Columbia Nursing’s Topaz explains why nurses need to understand AI, and how AI tools can help—and hurt—patient care.
Maxim Topaz, PhD, the Elizabeth Standish Gill Associate Professor of Nursing, conducts cutting-edge research with the goal of creating tools to help nurses provide the best possible care. For example, he and his colleagues built open-source, natural language processing software that allows clinicians and researchers to “mine” millions of patient records for patterns that could help health care providers identify at-risk patients.
Working with Columbia Nursing’s Professor Suzanne Bakken, PhD; Assistant Professor Kenrick Cato, PhD; and colleagues in Finland and China, Topaz organized a think-tank of the Nursing and Artificial Intelligence Leadership Collaborative (NAIL), which was held in October 2019. He was senior author of the report on the group’s conclusions and recommendations published May 18, 2021, in the Journal of Advanced Nursing.
Q: What is AI, and what are the most important things for nurses and nursing students to know about it?
A: By artificial intelligence, or AI, we mean any type of technology that can make intelligent decisions informed by a diverse range of data inputs. In health care, AI usually presents in the form of computer software or an application that helps clinicians to make better decisions about care they provide to their patients. AI is a tool that is rapidly advancing our ability to help patients, similar to other technological advances made over the past decades, like the invention of stethoscope or ultrasound. AI is particularly good at analyzing large quantities of patient data and identifying patterns humans might miss.
Q: What do Columbia Nursing students learn about AI?
A: At Columbia Nursing, we have some of the world’s leading researchers studying nursing-specific AI technologies. Students are exposed through their educational programs and research experiences to some of the most cutting-edge AI learning opportunities. For example, we just developed a course called “Data science for better patient outcomes: a nursing perspective.” The course is interdisciplinary, including students from AI-related fields (like engineering and computer science), and it provides hands-on opportunities for nurses to collaborate on creating AI systems of the future.
Q: Why is it essential for nurses to be involved in developing health care AI?
A: AI is becoming ubiquitous in health care and AI-based systems are being used by nurses and other clinicians in their everyday practice. Just as with understanding how the stethoscope works and its limitations, nurses have to understand how this new technology works.
Q: Are nurses using AI tools in the workplace today? If so, what do these tools look like?
A: AI is very common in current clinical practice. For example, electronic health records that are being used by virtually all nurses include several key AI-based clinical decision support tools. These tools range from alerts and alarms for abnormal vital signs to automated systems that predict a patient’s risk of death or rehospitalization.
Q: What are the most promising applications of AI for helping nurses take care of patients? What about for nursing research?
A: From my perspective, the most promising AI technologies are those that will help nurses to identify patients who are deteriorating during the course of their care. Nurses and other health care providers collect millions of data points about their patients, including vital signs, description of symptoms, lab findings, imaging results, etc. It is virtually impossible for a clinician to read through hundreds of pages in the patient chart and find certain deterioration patterns. AI technologies can actually help to process millions of data points and find patterns related to deterioration. At Columbia Nursing, we have several ongoing studies that use AI to identify patients who are deteriorating in hospitals and outpatient settings, such as home health care.
Q: What are the risks of using AI in health care? Are there risks that are unique to nursing?
A: AI can introduce several risks. First, AI can systematically embed existing human biases into electronic systems. For example, recently a clinical decision algorithm introduced racial bias by prioritizing care for less sick white patients over sicker Black patients in the U.S. In addition, AI tools can exacerbate the push towards market-driven goals of efficiency. For example, while certain AI technologies (e.g., voice recognition and automated transcription) can help nurses to reduce their documentation time, this newly freed-up time should not be allocated towards increasing the volume of patients and tasks assigned to nurses.
To address these risks, the NAIL collaborative recommended that nurses must understand the relationship between the data they collect and AI technologies they use, and be meaningfully involved in all stages of AI, from development to implementation.
There is a substantial untapped and unexplored potential for nursing to contribute to the development of AI technologies for global health and humanitarian efforts. Overall, we push for “AI for good nursing”!
Q: Tell me about the research you are currently working on.
A: As I mentioned, we have several studies where we are identifying patient deterioration using millions of data points collected about patients in electronic health records. Currently, we are also collaborating with the largest not-for-profit home health care organization in the U.S., the Visiting Nurse Service of New York, and the company Amazon on understanding the role of speech recognition technology in identifying patients at risk for hospitalization or emergency department visits. We also have a large clinical trial where we are using AI to help home health care nurses prioritize their incoming patients. Lastly, we have several ongoing studies examining the risk that AI systems may be affected by racial and/or other types of biases and identifying ways to reduce these biases.